Presented By: Department of Statistics Dissertation Defenses
Thesis Defense: Robust and Computationally Efficient Methods for High-Throughput Drug Screening Studies
Zoe Rehnberg
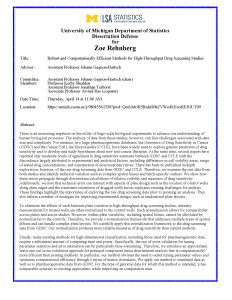
Abstract: There is an increasing emphasis on the utility of large-scale biological experiments to advance our understanding of human biological processes. The analysis of data from these studies, however, can face challenges associated with data size and complexity. For instance, two large pharmacogenomic databases, the Genomics of Drug Sensitivity in Cancer (GDSC) and the Cancer Cell Line Encyclopedia (CCLE), have been widely used to explore genetic predictors of drug sensitivity and to develop and study hypotheses about new anti-cancer therapies. At the same time, several papers have reported only moderate levels of agreement in drug sensitivity estimates between GDSC and CCLE with the discordance largely attributed to experimental and analytical factors, including differences in cell viability assay, range of tested drug concentrations, and construction of dose-response curves. There has been no published in-depth exploration, however, of the raw drug screening data from GDSC and CCLE. Therefore, we examine the raw data from both studies and identify technical variation such as complex spatial biases and batch-specific outliers. We show how these errors propagate through downstream calculations of relative viability and measures of drug sensitivity. Additionally, we note that technical error can interact with aspects of plate design such as the location of control wells along plate edges and the consistent orientation of drugged wells across replicates creating challenges for analysis. These findings highlight the importance of exploring the raw drug screening data prior to pursuing an analysis. They also inform a number of strategies for improving experimental design, such as randomized plate layouts.
To eliminate the effects of such between-plate variation in high-throughput drug screening studies, intensity measurements for treated wells are often normalized to the control wells. Such normalization allows for comparability across plates and across studies. However, within-plate variability, including spatial biases, cannot be alleviated by normalization to the controls. Therefore, we provide a normalization framework that addresses multiple types of spatial effects and can handle complex plate layouts. We carefully apply this normalization framework to the drug screening data from GDSC. Our normalization produces more reliable measures of drug sensitivity than current methods.
Finally, many existing methods for high-dimensional classification, including those used for pharmacogenomic data, require a substantial amount of computing time and power. Specifically, the use of cross-validation for tuning parameter selection and error estimation can be particularly time-consuming. Therefore, we introduce an approximate leave-one-out cross-validation approach for principal component linear discriminant analysis that is computationally more efficient than existing methods. In particular, our method obviates the need to select tuning parameter values and optimizes computational efficiency through a series of matrix downdates. We apply our method to simulated data as well as to pharmacogenomic data from GDSC. For the type of genomic data for which this method is intended, it has comparable accuracy to existing approaches, while improving on computation time.
To eliminate the effects of such between-plate variation in high-throughput drug screening studies, intensity measurements for treated wells are often normalized to the control wells. Such normalization allows for comparability across plates and across studies. However, within-plate variability, including spatial biases, cannot be alleviated by normalization to the controls. Therefore, we provide a normalization framework that addresses multiple types of spatial effects and can handle complex plate layouts. We carefully apply this normalization framework to the drug screening data from GDSC. Our normalization produces more reliable measures of drug sensitivity than current methods.
Finally, many existing methods for high-dimensional classification, including those used for pharmacogenomic data, require a substantial amount of computing time and power. Specifically, the use of cross-validation for tuning parameter selection and error estimation can be particularly time-consuming. Therefore, we introduce an approximate leave-one-out cross-validation approach for principal component linear discriminant analysis that is computationally more efficient than existing methods. In particular, our method obviates the need to select tuning parameter values and optimizes computational efficiency through a series of matrix downdates. We apply our method to simulated data as well as to pharmacogenomic data from GDSC. For the type of genomic data for which this method is intended, it has comparable accuracy to existing approaches, while improving on computation time.
Livestream Information
Sign In To View Joining InformationExplore Similar Events
-
Loading Similar Events...