Presented By: Department of Statistics Dissertation Defenses
Thesis Defense: An Accurate and Scalable Approach to Classifying High-Dimensional Data With Dense Latent Structure
Nora Yujia Payne
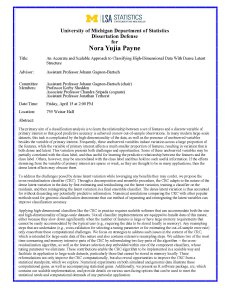
Abstract:
The primary aim of a classification analysis is to learn the relationship between a set of features and a discrete variable of primary interest so that good predictive accuracy is achieved on new out-of-sample observations. In many modern large-scale datasets, this task is complicated by the high-dimensionality of the data, as well as the presence of unobserved variables besides the variable of primary interest. Frequently, these unobserved variables induce variation across a large proportion of the features, while the variable of primary interest affects a much smaller proportion of features, resulting in variation that is both dense and latent. This variation presents both challenges and opportunities. Some of these unobserved variables may be partially correlated with the class label, and thus useful for learning the predictive relationship between the features and the class label. Others, however, may be uncorrelated with the class label and thus hold no such useful information. If the effects stemming from the variable of primary interest are sparse or weak, as they are thought to be in many applications, then the dense latent effects may obscure them.
To address the challenges posed by dense latent variation while leveraging any benefits they may confer, we propose the cross-residualization classifier (CRC). Through a decomposition and ensemble procedure, the CRC adapts to the nature of the dense latent variation in the data by first estimating and residualizing out the latent variation, training a classifier on the residuals, and then reintegrating the latent variation in a final ensemble classifier. The dense latent variation is thus accounted for without discarding any potentially predictive information. Numerical simulations comparing the CRC with other popular methods used for genomic classification demonstrate that our method of separating and reintegrating the latent variables can improve classification accuracy.
Applying high-dimensional classifiers like the CRC in practice requires scalable software that can accommodate both the size and high-dimensionality of large-scale datasets. Not all classifier implementations are equipped to handle data of this nature, either because they slow down significantly when the number of features is large or have large memory requirements that cannot be easily accommodated by the typical user (e.g., requiring the data to be stored locally in memory). Any resampling steps that are undertaken (e.g., cross-validation for selecting a tuning parameter or for estimating the out-of-sample error rate) only exacerbate these computational challenges. We focus on strategies to address such issues in the context of the CRC, which is intended for large-scale data of this nature and also contains extensive resampling steps. We address two of the most time-consuming and memory intensive parts of the CRC by reformulating two key parts of the algorithm -- the cross residualization algorithm, as well as the feature selection step embedded within one of the component classifiers, whose tuning parameter we eliminate. These contributions enable the CRC algorithm to be implemented in a scalable way and facilitate its application to large-scale datasets, particularly those that cannot be stored in memory locally. These reformulations not only improve the CRC computationally, but also reveal opportunities to improve the CRC from a statistical standpoint, which we explore. Numerical experiments on both simulated and genomic data illustrate these computational gains, as well as accompanying statistical gains. Additionally, we present an R software package, crc, which contains our scalable implementation, and provide details on various user-facing options that can be used to meet the statistical needs and computational demands of any particular application.
The primary aim of a classification analysis is to learn the relationship between a set of features and a discrete variable of primary interest so that good predictive accuracy is achieved on new out-of-sample observations. In many modern large-scale datasets, this task is complicated by the high-dimensionality of the data, as well as the presence of unobserved variables besides the variable of primary interest. Frequently, these unobserved variables induce variation across a large proportion of the features, while the variable of primary interest affects a much smaller proportion of features, resulting in variation that is both dense and latent. This variation presents both challenges and opportunities. Some of these unobserved variables may be partially correlated with the class label, and thus useful for learning the predictive relationship between the features and the class label. Others, however, may be uncorrelated with the class label and thus hold no such useful information. If the effects stemming from the variable of primary interest are sparse or weak, as they are thought to be in many applications, then the dense latent effects may obscure them.
To address the challenges posed by dense latent variation while leveraging any benefits they may confer, we propose the cross-residualization classifier (CRC). Through a decomposition and ensemble procedure, the CRC adapts to the nature of the dense latent variation in the data by first estimating and residualizing out the latent variation, training a classifier on the residuals, and then reintegrating the latent variation in a final ensemble classifier. The dense latent variation is thus accounted for without discarding any potentially predictive information. Numerical simulations comparing the CRC with other popular methods used for genomic classification demonstrate that our method of separating and reintegrating the latent variables can improve classification accuracy.
Applying high-dimensional classifiers like the CRC in practice requires scalable software that can accommodate both the size and high-dimensionality of large-scale datasets. Not all classifier implementations are equipped to handle data of this nature, either because they slow down significantly when the number of features is large or have large memory requirements that cannot be easily accommodated by the typical user (e.g., requiring the data to be stored locally in memory). Any resampling steps that are undertaken (e.g., cross-validation for selecting a tuning parameter or for estimating the out-of-sample error rate) only exacerbate these computational challenges. We focus on strategies to address such issues in the context of the CRC, which is intended for large-scale data of this nature and also contains extensive resampling steps. We address two of the most time-consuming and memory intensive parts of the CRC by reformulating two key parts of the algorithm -- the cross residualization algorithm, as well as the feature selection step embedded within one of the component classifiers, whose tuning parameter we eliminate. These contributions enable the CRC algorithm to be implemented in a scalable way and facilitate its application to large-scale datasets, particularly those that cannot be stored in memory locally. These reformulations not only improve the CRC computationally, but also reveal opportunities to improve the CRC from a statistical standpoint, which we explore. Numerical experiments on both simulated and genomic data illustrate these computational gains, as well as accompanying statistical gains. Additionally, we present an R software package, crc, which contains our scalable implementation, and provide details on various user-facing options that can be used to meet the statistical needs and computational demands of any particular application.
Explore Similar Events
-
Loading Similar Events...