Presented By: Department of Statistics Dissertation Defenses
Statistical Learning for Latent Attribute Models
Chenchen Ma
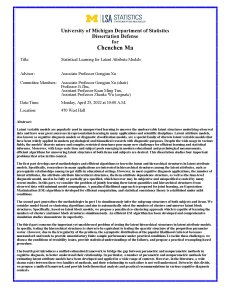
Abstract:
Latent variable models are popularly used in unsupervised learning to uncover the unobservable latent structures underlying observed data and have seen great successes in representation learning in many applications and scientific disciplines. Latent attribute models, also known as cognitive diagnosis models or diagnostic classification models, are a special family of discrete latent variable models that have been widely applied in modern psychological and biomedical research with diagnostic purposes. Despite the wide usage in various fields, the models' discrete nature and complex restricted structures pose many new challenges for efficient learning and statistical inference. Moreover, with large-scale item and subject pools emerging in modern educational and psychological measurements, efficient algorithms for uncovering latent structures of both items and subjects are desired. This dissertation studies four important problems that arise in this context.
The first part develops novel methodologies and efficient algorithms to learn the latent and hierarchical structures in latent attribute models. Specifically, researchers in many applications are interested in hierarchical structures among the latent attributes, such as prerequisite relationships among target skills in educational settings. However, in most cognitive diagnosis applications, the number of latent attributes, the attribute-attribute hierarchical structures, the item-attribute dependence structure, as well as the item-level diagnostic model, need to be fully or partially pre-specified, which however may be subjective and misspecified as noted by many recent studies. In this part, we consider the problem of jointly learning these latent quantities and hierarchical structures from observed data with minimal model assumptions. A penalized likelihood approach is proposed for joint learning, an Expectation Maximization (EM) algorithm is developed for efficient computation, and statistical consistency theory is established under mild conditions.
The second part generalizes the methodologies in part I to simultaneously infer the subgroup structures of both subjects and items. We consider model-based co-clustering algorithms and aim to automatically select the numbers of clusters and uncover latent block structures. Specifically, based on latent block models, we propose a penalized co-clustering approach which is capable of learning the numbers of clusters and inner block structures simultaneously. An efficient EM algorithm has been developed and comprehensive simulation studies demonstrate its superiority.
The third part concerns the important yet unaddressed problem of testing the latent hierarchical structures in latent attribute models. In specific, testing the hierarchical structures is shown to be equivalent to testing the sparsity structure of the proportion parameter vector. However, due to the irregularity of the problem, the asymptotic distribution of the popular likelihood ratio test becomes nonstandard and tends to provide unsatisfactory finite sample performance under practical conditions.To tackle these challenges, we discuss the conditions of testability issues, provide statistical understandings of the failures, and propose a practical resampling-based procedure.
The fourth part introduces a unified estimation framework to bridge the gap between parametric and nonparametric methods in cognitive diagnosis, to better understand their relationship. In particular, a number of parametric and nonparametric methods for estimating latent attribute models have been developed and applied in a wide range of contexts. However, in the literature, a wide chasm exists between these two families of methods, and their relationship to each other is not well understood. Driven by this divide, we propose a unified framework and provide both theoretical analysis and practical recommendations in various cognitive diagnosis contexts.
Latent variable models are popularly used in unsupervised learning to uncover the unobservable latent structures underlying observed data and have seen great successes in representation learning in many applications and scientific disciplines. Latent attribute models, also known as cognitive diagnosis models or diagnostic classification models, are a special family of discrete latent variable models that have been widely applied in modern psychological and biomedical research with diagnostic purposes. Despite the wide usage in various fields, the models' discrete nature and complex restricted structures pose many new challenges for efficient learning and statistical inference. Moreover, with large-scale item and subject pools emerging in modern educational and psychological measurements, efficient algorithms for uncovering latent structures of both items and subjects are desired. This dissertation studies four important problems that arise in this context.
The first part develops novel methodologies and efficient algorithms to learn the latent and hierarchical structures in latent attribute models. Specifically, researchers in many applications are interested in hierarchical structures among the latent attributes, such as prerequisite relationships among target skills in educational settings. However, in most cognitive diagnosis applications, the number of latent attributes, the attribute-attribute hierarchical structures, the item-attribute dependence structure, as well as the item-level diagnostic model, need to be fully or partially pre-specified, which however may be subjective and misspecified as noted by many recent studies. In this part, we consider the problem of jointly learning these latent quantities and hierarchical structures from observed data with minimal model assumptions. A penalized likelihood approach is proposed for joint learning, an Expectation Maximization (EM) algorithm is developed for efficient computation, and statistical consistency theory is established under mild conditions.
The second part generalizes the methodologies in part I to simultaneously infer the subgroup structures of both subjects and items. We consider model-based co-clustering algorithms and aim to automatically select the numbers of clusters and uncover latent block structures. Specifically, based on latent block models, we propose a penalized co-clustering approach which is capable of learning the numbers of clusters and inner block structures simultaneously. An efficient EM algorithm has been developed and comprehensive simulation studies demonstrate its superiority.
The third part concerns the important yet unaddressed problem of testing the latent hierarchical structures in latent attribute models. In specific, testing the hierarchical structures is shown to be equivalent to testing the sparsity structure of the proportion parameter vector. However, due to the irregularity of the problem, the asymptotic distribution of the popular likelihood ratio test becomes nonstandard and tends to provide unsatisfactory finite sample performance under practical conditions.To tackle these challenges, we discuss the conditions of testability issues, provide statistical understandings of the failures, and propose a practical resampling-based procedure.
The fourth part introduces a unified estimation framework to bridge the gap between parametric and nonparametric methods in cognitive diagnosis, to better understand their relationship. In particular, a number of parametric and nonparametric methods for estimating latent attribute models have been developed and applied in a wide range of contexts. However, in the literature, a wide chasm exists between these two families of methods, and their relationship to each other is not well understood. Driven by this divide, we propose a unified framework and provide both theoretical analysis and practical recommendations in various cognitive diagnosis contexts.
Explore Similar Events
-
Loading Similar Events...