Presented By: Department of Statistics Dissertation Defenses
Statistical Estimation and Inference for Large-Scale Categorical Data
Chengcheng Li
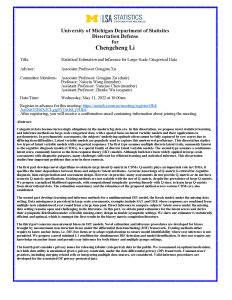
Abstract:
Categorical data become increasingly ubiquitous in the modern big data era. In this dissertation, we propose novel statistical learning and inference methods on large-scale categorical data, with a special focus on latent variable models and their applications to psychometrics. In psychometric assessments, the subjects' underlying aptitude often cannot be fully captured by raw scores due to differing item difficulties. Latent variable models are popularly used to capture this unobserved proficiency. This dissertation studies two types of latent variable models with categorical responses. The first type assumes multiple discrete latent traits, commonly known as the cognitive diagnosis models (CDMs), is a special family of discrete latent variable models. The second type assumes a continuous latent score, commonly known as the item response theory (IRT) models. Although both have been widely applied in large-scale assessments with diagnostic purposes, many challenges still exist for efficient learning and statistical inference. This dissertation studies four important problems that arise in these contexts.
The first part develops novel algorithms to estimate large latent Q-matrix in CDMs. Q-matrix plays an important role in CDMs; it specifies the inter-dependence between items and subjects' latent attributes. Accurate knowledge of Q-matrix is critical for cognitive diagnosis, item categorization and assessment design. However, in practice, many assessments do not provide Q-matrix or do not have accurate Q-matrix specifications. Existing methods are not scalable with the size of Q-matrix, despite the prevalence of large Q-matrix. We propose a penalized likelihood approach, with computational complexity growing linearly with Q sizes, to learn large Q-matrix from observational data. The estimation consistency and the robustness of the proposed method across various CDMs are also established.
The second part develops learning and inference methods for a unidimensional IRT model, the Rasch model, under the missing data setting. Data missingness is prevalent in large-scale assessments; examples include SAT and GRE where responses are combined from multiple tests administered year round from a large item pool. Direct inference to compare subjects’ latent scores under the missing data setting remains open and challenging in the literature. In this part, we obtain point estimators for the latent scores and derive their asymptotic distribution under a flexible missing-entry design in double asymptotic settings. We show our estimator is statistically efficient and optimal, which is amongst the first results in the binary matrix completion literature.
The third part concerns measurement biases in IRT models. Novel estimation and inference procedures are developed for biases brought by measurement non-invariant items under the differential item functioning (DIF) framework. Existing methods either require to know anchor items, i.e. DIF-free items or to adopt regularization to ensure model identifiability where easy inference is not permitted. We propose a novel minimal L1 condition for simultaneous DIF detection and model identification. It does not require any knowledge on anchor items and permits easy inference for both binary and multiple groups settings.
The fourth part considers privacy issues for releasing tabular (categorical) data to the public. We recommend an optimal mechanism, in which data utility is maximized given a privacy constraint, under the data differential privacy (DP) framework. Common users' practices, including merging related cells or integrating multiple data sources, are considered. Valid inference procedures are developed for the associated DP privacy-protected data.
Categorical data become increasingly ubiquitous in the modern big data era. In this dissertation, we propose novel statistical learning and inference methods on large-scale categorical data, with a special focus on latent variable models and their applications to psychometrics. In psychometric assessments, the subjects' underlying aptitude often cannot be fully captured by raw scores due to differing item difficulties. Latent variable models are popularly used to capture this unobserved proficiency. This dissertation studies two types of latent variable models with categorical responses. The first type assumes multiple discrete latent traits, commonly known as the cognitive diagnosis models (CDMs), is a special family of discrete latent variable models. The second type assumes a continuous latent score, commonly known as the item response theory (IRT) models. Although both have been widely applied in large-scale assessments with diagnostic purposes, many challenges still exist for efficient learning and statistical inference. This dissertation studies four important problems that arise in these contexts.
The first part develops novel algorithms to estimate large latent Q-matrix in CDMs. Q-matrix plays an important role in CDMs; it specifies the inter-dependence between items and subjects' latent attributes. Accurate knowledge of Q-matrix is critical for cognitive diagnosis, item categorization and assessment design. However, in practice, many assessments do not provide Q-matrix or do not have accurate Q-matrix specifications. Existing methods are not scalable with the size of Q-matrix, despite the prevalence of large Q-matrix. We propose a penalized likelihood approach, with computational complexity growing linearly with Q sizes, to learn large Q-matrix from observational data. The estimation consistency and the robustness of the proposed method across various CDMs are also established.
The second part develops learning and inference methods for a unidimensional IRT model, the Rasch model, under the missing data setting. Data missingness is prevalent in large-scale assessments; examples include SAT and GRE where responses are combined from multiple tests administered year round from a large item pool. Direct inference to compare subjects’ latent scores under the missing data setting remains open and challenging in the literature. In this part, we obtain point estimators for the latent scores and derive their asymptotic distribution under a flexible missing-entry design in double asymptotic settings. We show our estimator is statistically efficient and optimal, which is amongst the first results in the binary matrix completion literature.
The third part concerns measurement biases in IRT models. Novel estimation and inference procedures are developed for biases brought by measurement non-invariant items under the differential item functioning (DIF) framework. Existing methods either require to know anchor items, i.e. DIF-free items or to adopt regularization to ensure model identifiability where easy inference is not permitted. We propose a novel minimal L1 condition for simultaneous DIF detection and model identification. It does not require any knowledge on anchor items and permits easy inference for both binary and multiple groups settings.
The fourth part considers privacy issues for releasing tabular (categorical) data to the public. We recommend an optimal mechanism, in which data utility is maximized given a privacy constraint, under the data differential privacy (DP) framework. Common users' practices, including merging related cells or integrating multiple data sources, are considered. Valid inference procedures are developed for the associated DP privacy-protected data.
Explore Similar Events
-
Loading Similar Events...