Presented By: Biomedical Engineering
Data-driven Methods for Automated Assessment of Coronary Artery Disease
BME Ph.D. Defense: Kritika Iyer
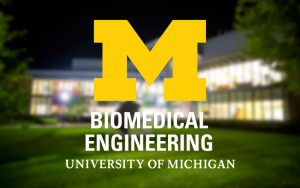
Abstract:
The current gold standard for Coronary Artery Disease (CAD) diagnosis is X-ray angiography. Visual estimation can be subjective, therefore semi-automated software tools such as Quantitative Coronary Angiography (QCA) have been developed to quantify disease severity. Alternatively, functional metrics such as Fractional Flow Reserve (FFR) have demonstrated better diagnostic outcomes than anatomical assessment, but they are not widely used due to cost and risk. Ideally, quantitative and functional information could be derived directly from X-ray angiography images without the additional risks, time, and cost associated with performing FFR or QCA.
The goal of this project is to develop automated data-driven approaches for anatomical and functional quantification of disease severity using X-ray angiography images. To this end, we have developed algorithms for 1) automated coronary vessel segmentation, 2) stenosis detection and characterization, 3) 3D reconstruction of coronary anatomy, and 4) image-based flow extraction. These algorithms can be used in conjunction with computational fluid dynamics (CFD) modeling to assess the functional significance of disease.
We first present AngioNet, a neural network for coronary segmentation from X-ray angiography images. Conventional algorithms relying on thresholding or filtering cannot distinguish between the coronary vessels and the catheter used to inject the dye. AngioNet’s key innovation is an Angiographic Processing Network, or APN, which learns the best possible combination of pre-processing filters to improve segmentation performance. AngioNet demonstrates state-of-the-art segmentation accuracy (Dice score = 0.864) and does not segment the catheter in challenging cases where other neural networks fail.
Building upon AngioNet, we developed combination of neural networks and image processing algorithms to automatically localize, segment, and measure stenoses. This pipeline was able to measure stenosis diameter within 0.206±0.155mm or approximately 1 pixel of ground truth measurements from QCA. It is also the first automated pipeline to quantify rather than categorize disease severity.
Although measuring stenosis diameter in 2D images is useful, a more robust approach would be to measure diameters in the 3D coronary anatomy. Another advantage of the 3D coronary anatomy is that it can be used to perform CFD simulations of blood flow and compute functional metrics such as FFR. To this end, we developed a machine learning approach for automated 3D vessel reconstruction from a series of uncalibrated 2D X-ray angiography images. This approach is superior to projective geometry methods for 3D reconstruction due to their semi-automatic nature and reliance on accurate knowledge of input image acquisition angles. Our machine learning approach has demonstrated sub-pixel error in radius reconstruction (0.16±0.07mm) and 1% error in FFR computed in a reconstructed coronary tree.
In addition to the 3D coronary geometry, information about patient-specific flow or pressure is required to perform a hemodynamics simulation and compute FFR. We developed an algorithm that tracks vessel area in sequential frames of a segmented angiography series to estimate relative flow in each branch. We validated the algorithm in the simplest possible case, using a simulation of dye transport under steady flow conditions as the ground truth. On average, the difference in relative flow per branch was 5.15% for a healthy coronary tree and 3.68% in a coronary tree with stenosis.
We finally demonstrated the successes and limitations of the methods developed in this thesis by comparing computational FFR derived using the above algorithms against clinically measured FFR. The error between the calculated and clinically measured FFR was 0.1, corresponding to an 11% error.
Committee Chair(s):
Dr. C. Alberto Figueroa and Dr. Brahmajee K. Nallamothu
Zoom Link: https://umich.zoom.us/j/94364657250, Passcode: 390041 *Registration is required
The current gold standard for Coronary Artery Disease (CAD) diagnosis is X-ray angiography. Visual estimation can be subjective, therefore semi-automated software tools such as Quantitative Coronary Angiography (QCA) have been developed to quantify disease severity. Alternatively, functional metrics such as Fractional Flow Reserve (FFR) have demonstrated better diagnostic outcomes than anatomical assessment, but they are not widely used due to cost and risk. Ideally, quantitative and functional information could be derived directly from X-ray angiography images without the additional risks, time, and cost associated with performing FFR or QCA.
The goal of this project is to develop automated data-driven approaches for anatomical and functional quantification of disease severity using X-ray angiography images. To this end, we have developed algorithms for 1) automated coronary vessel segmentation, 2) stenosis detection and characterization, 3) 3D reconstruction of coronary anatomy, and 4) image-based flow extraction. These algorithms can be used in conjunction with computational fluid dynamics (CFD) modeling to assess the functional significance of disease.
We first present AngioNet, a neural network for coronary segmentation from X-ray angiography images. Conventional algorithms relying on thresholding or filtering cannot distinguish between the coronary vessels and the catheter used to inject the dye. AngioNet’s key innovation is an Angiographic Processing Network, or APN, which learns the best possible combination of pre-processing filters to improve segmentation performance. AngioNet demonstrates state-of-the-art segmentation accuracy (Dice score = 0.864) and does not segment the catheter in challenging cases where other neural networks fail.
Building upon AngioNet, we developed combination of neural networks and image processing algorithms to automatically localize, segment, and measure stenoses. This pipeline was able to measure stenosis diameter within 0.206±0.155mm or approximately 1 pixel of ground truth measurements from QCA. It is also the first automated pipeline to quantify rather than categorize disease severity.
Although measuring stenosis diameter in 2D images is useful, a more robust approach would be to measure diameters in the 3D coronary anatomy. Another advantage of the 3D coronary anatomy is that it can be used to perform CFD simulations of blood flow and compute functional metrics such as FFR. To this end, we developed a machine learning approach for automated 3D vessel reconstruction from a series of uncalibrated 2D X-ray angiography images. This approach is superior to projective geometry methods for 3D reconstruction due to their semi-automatic nature and reliance on accurate knowledge of input image acquisition angles. Our machine learning approach has demonstrated sub-pixel error in radius reconstruction (0.16±0.07mm) and 1% error in FFR computed in a reconstructed coronary tree.
In addition to the 3D coronary geometry, information about patient-specific flow or pressure is required to perform a hemodynamics simulation and compute FFR. We developed an algorithm that tracks vessel area in sequential frames of a segmented angiography series to estimate relative flow in each branch. We validated the algorithm in the simplest possible case, using a simulation of dye transport under steady flow conditions as the ground truth. On average, the difference in relative flow per branch was 5.15% for a healthy coronary tree and 3.68% in a coronary tree with stenosis.
We finally demonstrated the successes and limitations of the methods developed in this thesis by comparing computational FFR derived using the above algorithms against clinically measured FFR. The error between the calculated and clinically measured FFR was 0.1, corresponding to an 11% error.
Committee Chair(s):
Dr. C. Alberto Figueroa and Dr. Brahmajee K. Nallamothu
Zoom Link: https://umich.zoom.us/j/94364657250, Passcode: 390041 *Registration is required