Presented By: Michigan Program in Survey and Data Science
CANCELLED - MPSDS JPSM Seminar Series - Using Synergies Between Survey Statistics and Causal Inference to Improve Transportability of Clinical Trials
CANCELLED - Michael Elliott - Survey Methodology Program - Michigan Program in Survey and Data Science
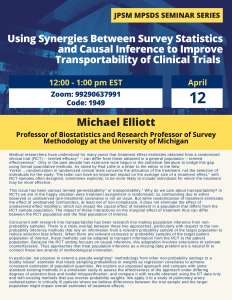
CANCELLED
MPSDS JPSM Seminar Series
April 12, 2023
12:00 - 1:00 EST
Michael Elliott is a Professor of Biostatistics and a Research Professor at the Institute for Social Research. Dr. Elliott's research interests include the design and analysis of sample surveys, causal inference, and missing and latent variable data structures.
Abstract
Medical researchers have understood for many years that treatment effect estimates obtained from a randomized clinical trial (RCT) -- termed efficacy'' -- can differ from those obtained in a general population -- termed effectiveness''. Only in the past decade has extensive work begun in the statistical literature to bridge this gap using formal quantitative methods. As noted by Rod Little in a letter to the editor in the New Yorker ...randomization in randomized clinical trials concerns the allocation of the treatment, not the selection of individuals for the study. The latter can have an important impact on the average size of a treatment effect,'' with RCT samples often designed, sometimes explicitly, to be more likely to include individuals for whom the treatment may be more effective.
This issue has been various termed generalizability'' or transportability." Why do we care about transportability? In RCTs we are in the happy situation were treatment assignment is randomized, so confounding due to either observed or unobserved (pre-treatment) covariates is not an issue. But while randomization of treatment eliminates the effect of unobserved confounders, at least net of non-compliance, it does not eliminate the effect of unobserved effect modifiers, which can impact the causal effect of treatment in a population that differs from the RCT sample population. The impact of these interactions on the marginal effect of treatment thus can differ between the RCT population and the final population of interest.
Concurrent with research into transportability has been research into making population inference from non-probability samples. There is a close overlap between these two approaches, particularly with respect to the non-probability inference methods that rely on information from a relevant probability sample of the target population to reduce selection bias effects. When there are relevant censuses or probability samples of the target patient population of interest, these methods can be adapted to transport information from the RCT to the patient population. Because the RCT setting focuses on causal inference, this adaptation involves extensions to estimate counterfactuals. Thus approaches that treat population inference as a missing data problem are a natural fit to connect these two strands of methodological innovation.
In particular, we propose to extend a pseudo-weighting'' methodology from other non-probability settings to a doubly robust'' estimator that treats sampling probabilities or weights as regression covariates to achieve consistent estimation of population quantities. We explore our proposed approach and compare with some standard existing methods in a simulation study to assess the effectiveness of the approach under differing degrees of selection bias and model misspecification, and compare it with results obtained using the RT data only and with existing methods that use inverse probability weights. We apply it to a study of pulmonary artery catheterization in critically ill patients where we believe differences between the trial sample and the larger population might impact overall estimates of treatment effects.
MPSDS
The University of Michigan Program in Survey Methodology was established in 2001 seeking to train future generations of survey and data scientists. In 2021, we changed our name to the Michigan Program in Survey and Data Science. Our curriculum is concerned with a broad set of data sources including survey data, but also including social media posts, sensor data, and administrative records, as well as analytic methods for working with these new data sources. And we bring to data science a focus on data quality — which is not at the center of traditional data science. The new name speaks to what we teach and work on at the intersection of social research and data. The program offers doctorate and master of science degrees and a certificate through the University of Michigan. The program's home is the Institute for Social Research, the world's largest academically-based social science research institute.
Summer Institute in Survey Research Techniques (SISRT)
The mission of the Summer Institute is to provide rigorous and high quality graduate training in all phases of survey research. The program teaches state-of-the-art practice and theory in the design, implementation, and analysis of surveys. The Summer Institute in Survey Research Techniques has presented courses on the sample survey since the summer of 1948, and has offered such courses every summer since. Graduate-level courses through the Program in Survey and Data Science are offered from June 5 through July 28 and available to enroll in as a Summer Scholar.
The Summer Institute uses the sample survey as the basic instrument for the scientific measurement of human activity. It presents sample survey methods in courses designed to meet the educational needs of those specializing in social and behavioral research such as professionals in business, public health, natural resources, law, medicine, nursing, social work, and many other domains of study.
MPSDS JPSM Seminar Series
April 12, 2023
12:00 - 1:00 EST
Michael Elliott is a Professor of Biostatistics and a Research Professor at the Institute for Social Research. Dr. Elliott's research interests include the design and analysis of sample surveys, causal inference, and missing and latent variable data structures.
Abstract
Medical researchers have understood for many years that treatment effect estimates obtained from a randomized clinical trial (RCT) -- termed efficacy'' -- can differ from those obtained in a general population -- termed effectiveness''. Only in the past decade has extensive work begun in the statistical literature to bridge this gap using formal quantitative methods. As noted by Rod Little in a letter to the editor in the New Yorker ...randomization in randomized clinical trials concerns the allocation of the treatment, not the selection of individuals for the study. The latter can have an important impact on the average size of a treatment effect,'' with RCT samples often designed, sometimes explicitly, to be more likely to include individuals for whom the treatment may be more effective.
This issue has been various termed generalizability'' or transportability." Why do we care about transportability? In RCTs we are in the happy situation were treatment assignment is randomized, so confounding due to either observed or unobserved (pre-treatment) covariates is not an issue. But while randomization of treatment eliminates the effect of unobserved confounders, at least net of non-compliance, it does not eliminate the effect of unobserved effect modifiers, which can impact the causal effect of treatment in a population that differs from the RCT sample population. The impact of these interactions on the marginal effect of treatment thus can differ between the RCT population and the final population of interest.
Concurrent with research into transportability has been research into making population inference from non-probability samples. There is a close overlap between these two approaches, particularly with respect to the non-probability inference methods that rely on information from a relevant probability sample of the target population to reduce selection bias effects. When there are relevant censuses or probability samples of the target patient population of interest, these methods can be adapted to transport information from the RCT to the patient population. Because the RCT setting focuses on causal inference, this adaptation involves extensions to estimate counterfactuals. Thus approaches that treat population inference as a missing data problem are a natural fit to connect these two strands of methodological innovation.
In particular, we propose to extend a pseudo-weighting'' methodology from other non-probability settings to a doubly robust'' estimator that treats sampling probabilities or weights as regression covariates to achieve consistent estimation of population quantities. We explore our proposed approach and compare with some standard existing methods in a simulation study to assess the effectiveness of the approach under differing degrees of selection bias and model misspecification, and compare it with results obtained using the RT data only and with existing methods that use inverse probability weights. We apply it to a study of pulmonary artery catheterization in critically ill patients where we believe differences between the trial sample and the larger population might impact overall estimates of treatment effects.
MPSDS
The University of Michigan Program in Survey Methodology was established in 2001 seeking to train future generations of survey and data scientists. In 2021, we changed our name to the Michigan Program in Survey and Data Science. Our curriculum is concerned with a broad set of data sources including survey data, but also including social media posts, sensor data, and administrative records, as well as analytic methods for working with these new data sources. And we bring to data science a focus on data quality — which is not at the center of traditional data science. The new name speaks to what we teach and work on at the intersection of social research and data. The program offers doctorate and master of science degrees and a certificate through the University of Michigan. The program's home is the Institute for Social Research, the world's largest academically-based social science research institute.
Summer Institute in Survey Research Techniques (SISRT)
The mission of the Summer Institute is to provide rigorous and high quality graduate training in all phases of survey research. The program teaches state-of-the-art practice and theory in the design, implementation, and analysis of surveys. The Summer Institute in Survey Research Techniques has presented courses on the sample survey since the summer of 1948, and has offered such courses every summer since. Graduate-level courses through the Program in Survey and Data Science are offered from June 5 through July 28 and available to enroll in as a Summer Scholar.
The Summer Institute uses the sample survey as the basic instrument for the scientific measurement of human activity. It presents sample survey methods in courses designed to meet the educational needs of those specializing in social and behavioral research such as professionals in business, public health, natural resources, law, medicine, nursing, social work, and many other domains of study.
Explore Similar Events
-
Loading Similar Events...