Presented By: Michigan Program in Survey and Data Science
MPSDS JPSM Seminar Series - The Evolution of the Use of Models in Survey Sampling
Richard Valliant - Institute for Social Research - Joint Program in Survey Methodology
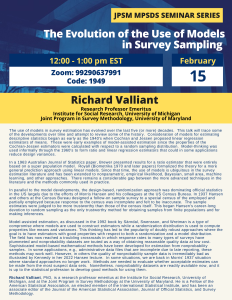
MPSDS JPSM Seminar Series
February 15, 2023
12:00 - 1:00 EST
Richard Valliant, PhD, is a research professor emeritus at the Institute for Social Research, University of Michigan, and at the Joint Program in Survey Methodology at the University of Maryland. He is a Fellow of the American Statistical Association, an elected member of the International Statistical Institute, and has been an associate editor of the Journal of the American Statistical Association, Journal of Official Statistics, and Survey Methodology.
The Evolution of the Use of Models in Survey Sampling
The use of models in survey estimation has evolved over the last five (or more) decades. This talk will trace some of the developments over time and attempt to review some of the history. Consideration of models for estimating descriptive statistics began as early as the 1940's when Cochran and Jessen proposed linear regression estimators of means. These were early examples of model-assisted estimation since the properties of the Cochran-Jessen estimators were calculated with respect to a random sampling distribution. Model-thinking was used informally through the 1960's to form ratio and linear regression estimators that could in some applications reduce design variances.
In a 1963 Australian Journal of Statistics paper, Brewer presented results for a ratio estimator that were entirely based on a super population model. Royall (Biometrika 1970 and later papers) formalized the theory for a more general prediction approach using linear models. Since that time, the use of models is ubiquitous in the survey estimation literature and has been extended to nonparametric, empirical likelihood, Bayesian, small area, machine learning, and other approaches. There remains a considerable gap between the more advanced techniques in the literature and the methods commonly used in practice.
In parallel to the model developments, the design-based, randomization approach was dominating official statistics in the US largely due to the efforts of Morris Hansen and his colleagues at the US Census Bureau. In 1937 Hansen and others at the Census Bureau designed a follow-on sample survey to a special census of the employed and partially employed because response to the census was incomplete and felt to be inaccurate. The sample estimates were judged to be more trustworthy than those of the census itself. This began Hansen’s career-long devotion to random sampling as the only trustworthy method for obtaining samples from finite populations and for making inferences.
Model-assisted estimation, as discussed in the 1992 book by Särndal, Swensson, and Wretman is a type of compromise where models are used to construct estimators while a randomization distribution is used to compute properties like means and variances. This thinking has led to the popularity of doubly robust approaches where the goal is to have estimators with good properties with respect to both a randomization and a model distribution.
The field has now reached a troubling crossroads in which response rates to many types of surveys have plummeted and nonprobability datasets are touted as a way of obtaining reasonable quality data at low cost. Sophisticated model-based mathematical methods have been developed for estimation from nonprobability samples. In some applications, e.g., administrative data files that are incomplete due to late reporting, these methods may work well. However, in others the quality of nonprobability sample data is irremediably bad as illustrated by Kennedy in her 2022 Hansen lecture. In some situations, we are back in Morris' 1937 situation where standard approaches no longer work. Methods are needed to evaluate whether acceptable estimates can be made from the most suspect data sets. Nonetheless. nonprobability datasets are readily available now, and it is up to the statistical profession to develop good methods for using them.
Michigan Program in Survey and Data Science (MPSDS)
The University of Michigan Program in Survey Methodology was established in 2001 seeking to train future generations of survey and data scientists. In 2021, we changed our name to the Michigan Program in Survey and Data Science. Our curriculum is concerned with a broad set of data sources including survey data, but also including social media posts, sensor data, and administrative records, as well as analytic methods for working with these new data sources. And we bring to data science a focus on data quality — which is not at the center of traditional data science. The new name speaks to what we teach and work on at the intersection of social research and data. The program offers doctorate and master of science degrees and a certificate through the University of Michigan. The program's home is the Institute for Social Research, the world's largest academically-based social science research institute.
Summer Institute in Survey Research Techniques (SISRT)
The mission of the Summer Institute is to provide rigorous and high quality graduate training in all phases of survey research. The program teaches state-of-the-art practice and theory in the design, implementation, and analysis of surveys. The Summer Institute in Survey Research Techniques has presented courses on the sample survey since the summer of 1948, and has offered such courses every summer since. Graduate-level courses through the Program in Survey and Data Science are offered from June 5 through July 28 and available to enroll in as a Summer Scholar.
February 15, 2023
12:00 - 1:00 EST
Richard Valliant, PhD, is a research professor emeritus at the Institute for Social Research, University of Michigan, and at the Joint Program in Survey Methodology at the University of Maryland. He is a Fellow of the American Statistical Association, an elected member of the International Statistical Institute, and has been an associate editor of the Journal of the American Statistical Association, Journal of Official Statistics, and Survey Methodology.
The Evolution of the Use of Models in Survey Sampling
The use of models in survey estimation has evolved over the last five (or more) decades. This talk will trace some of the developments over time and attempt to review some of the history. Consideration of models for estimating descriptive statistics began as early as the 1940's when Cochran and Jessen proposed linear regression estimators of means. These were early examples of model-assisted estimation since the properties of the Cochran-Jessen estimators were calculated with respect to a random sampling distribution. Model-thinking was used informally through the 1960's to form ratio and linear regression estimators that could in some applications reduce design variances.
In a 1963 Australian Journal of Statistics paper, Brewer presented results for a ratio estimator that were entirely based on a super population model. Royall (Biometrika 1970 and later papers) formalized the theory for a more general prediction approach using linear models. Since that time, the use of models is ubiquitous in the survey estimation literature and has been extended to nonparametric, empirical likelihood, Bayesian, small area, machine learning, and other approaches. There remains a considerable gap between the more advanced techniques in the literature and the methods commonly used in practice.
In parallel to the model developments, the design-based, randomization approach was dominating official statistics in the US largely due to the efforts of Morris Hansen and his colleagues at the US Census Bureau. In 1937 Hansen and others at the Census Bureau designed a follow-on sample survey to a special census of the employed and partially employed because response to the census was incomplete and felt to be inaccurate. The sample estimates were judged to be more trustworthy than those of the census itself. This began Hansen’s career-long devotion to random sampling as the only trustworthy method for obtaining samples from finite populations and for making inferences.
Model-assisted estimation, as discussed in the 1992 book by Särndal, Swensson, and Wretman is a type of compromise where models are used to construct estimators while a randomization distribution is used to compute properties like means and variances. This thinking has led to the popularity of doubly robust approaches where the goal is to have estimators with good properties with respect to both a randomization and a model distribution.
The field has now reached a troubling crossroads in which response rates to many types of surveys have plummeted and nonprobability datasets are touted as a way of obtaining reasonable quality data at low cost. Sophisticated model-based mathematical methods have been developed for estimation from nonprobability samples. In some applications, e.g., administrative data files that are incomplete due to late reporting, these methods may work well. However, in others the quality of nonprobability sample data is irremediably bad as illustrated by Kennedy in her 2022 Hansen lecture. In some situations, we are back in Morris' 1937 situation where standard approaches no longer work. Methods are needed to evaluate whether acceptable estimates can be made from the most suspect data sets. Nonetheless. nonprobability datasets are readily available now, and it is up to the statistical profession to develop good methods for using them.
Michigan Program in Survey and Data Science (MPSDS)
The University of Michigan Program in Survey Methodology was established in 2001 seeking to train future generations of survey and data scientists. In 2021, we changed our name to the Michigan Program in Survey and Data Science. Our curriculum is concerned with a broad set of data sources including survey data, but also including social media posts, sensor data, and administrative records, as well as analytic methods for working with these new data sources. And we bring to data science a focus on data quality — which is not at the center of traditional data science. The new name speaks to what we teach and work on at the intersection of social research and data. The program offers doctorate and master of science degrees and a certificate through the University of Michigan. The program's home is the Institute for Social Research, the world's largest academically-based social science research institute.
Summer Institute in Survey Research Techniques (SISRT)
The mission of the Summer Institute is to provide rigorous and high quality graduate training in all phases of survey research. The program teaches state-of-the-art practice and theory in the design, implementation, and analysis of surveys. The Summer Institute in Survey Research Techniques has presented courses on the sample survey since the summer of 1948, and has offered such courses every summer since. Graduate-level courses through the Program in Survey and Data Science are offered from June 5 through July 28 and available to enroll in as a Summer Scholar.
Livestream Information
ZoomFebruary 15, 2023 (Wednesday) 12:00pm
Meeting ID: 99290637991
Meeting Password: 1949
Explore Similar Events
-
Loading Similar Events...