Presented By: Michigan Robotics
Affordance-grounded Robot Perception and Manipulation in Adversarial, Translucent, and Cluttered Environments
PhD Defense, Xiaotong Chen
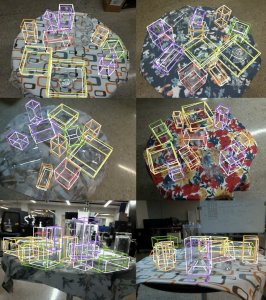
Chair: Chad Jenkins
Abstract:
Robots in the future need to work in natural scenarios and finish a variety of tasks without much supervision from humans. To achieve this goal, we want the robots to perform perception and action robustly and adaptively in unstructured environments. For example, robots are expected to correctly perceive objects in unseen cases, such as dark environments, heavy clutters or transparent materials. Besides, they should learn skills that are transferable across novel objects in categories rather than fixed on known instances. In this dissertation, we focus on the problem of perceiving and manipulating various objects in complex adversarial environments. Specifically, we explore on three aspects including robustness to adversarial environments, synergistic perception and action, and scalable data-driven perception pipelines for customized settings.
First, we explore the possibility to achieve robustness for object pose estimation algorithms against environmental changes, like object occlusions and lighting changes. We contribute a two-stage approach GRIP that combines both the discriminative power of deep convolutional neural networks (CNNs) and robustness in probabilistic generative inference. Our results indicate that GRIP has better accuracy through comparison with end-to-end pose estimation baselines, and efficacy in a grocery packing task in the dark scene.
Second, we focus on how to generalize object representation to category-level with grounded affordance for task execution. We propose the Affordance Coordinate Frame (ACF) representation that enables direct connection between perception and executable action. Along with that, an object part category-level scene perception pipeline is contributed to estimate ACFs in cluttered environments on novel objects. Our pipeline outperforms state-of-the-art methods for object detection, as well as category-level pose estimation for object parts. We further demonstrate the applicability of ACF to robot manipulation tasks like grasping, pouring and stirring.
Third, we contribute an annotation pipeline that enables large-scale dataset creation and benchmarking on transparent objects. The proposed ProgressLabeller pipeline has a multi-view annotation interface that allows fast and accurate pose annotation on RGB-D video streams. ProgressLabeller is proved to generate more accurate annotations in object pose estimation and grasping experiments. Using ProgressLabeller, we contributed ClearPose as the first large-scale RGB-D transparent object dataset with various adversarial conditions such as lighting changes, object clutters. ClearPose is made to support benchmarking of data-driven approaches on depth completion, object pose estimation and robotic manipulation. Then, we built an object pose estimation based manipulation framework, TransNet, for daily transparent objects. The system aims to generalize the pose estimation to unseen novel objects defined in several categories like wine cups and bottles. We finally demonstrate the efficacy of the system with robotic pick-and-place and pouring tasks, paving the way for more complex manipulations such as table-setting and drink serving.
Zoom password: 217944
Abstract:
Robots in the future need to work in natural scenarios and finish a variety of tasks without much supervision from humans. To achieve this goal, we want the robots to perform perception and action robustly and adaptively in unstructured environments. For example, robots are expected to correctly perceive objects in unseen cases, such as dark environments, heavy clutters or transparent materials. Besides, they should learn skills that are transferable across novel objects in categories rather than fixed on known instances. In this dissertation, we focus on the problem of perceiving and manipulating various objects in complex adversarial environments. Specifically, we explore on three aspects including robustness to adversarial environments, synergistic perception and action, and scalable data-driven perception pipelines for customized settings.
First, we explore the possibility to achieve robustness for object pose estimation algorithms against environmental changes, like object occlusions and lighting changes. We contribute a two-stage approach GRIP that combines both the discriminative power of deep convolutional neural networks (CNNs) and robustness in probabilistic generative inference. Our results indicate that GRIP has better accuracy through comparison with end-to-end pose estimation baselines, and efficacy in a grocery packing task in the dark scene.
Second, we focus on how to generalize object representation to category-level with grounded affordance for task execution. We propose the Affordance Coordinate Frame (ACF) representation that enables direct connection between perception and executable action. Along with that, an object part category-level scene perception pipeline is contributed to estimate ACFs in cluttered environments on novel objects. Our pipeline outperforms state-of-the-art methods for object detection, as well as category-level pose estimation for object parts. We further demonstrate the applicability of ACF to robot manipulation tasks like grasping, pouring and stirring.
Third, we contribute an annotation pipeline that enables large-scale dataset creation and benchmarking on transparent objects. The proposed ProgressLabeller pipeline has a multi-view annotation interface that allows fast and accurate pose annotation on RGB-D video streams. ProgressLabeller is proved to generate more accurate annotations in object pose estimation and grasping experiments. Using ProgressLabeller, we contributed ClearPose as the first large-scale RGB-D transparent object dataset with various adversarial conditions such as lighting changes, object clutters. ClearPose is made to support benchmarking of data-driven approaches on depth completion, object pose estimation and robotic manipulation. Then, we built an object pose estimation based manipulation framework, TransNet, for daily transparent objects. The system aims to generalize the pose estimation to unseen novel objects defined in several categories like wine cups and bottles. We finally demonstrate the efficacy of the system with robotic pick-and-place and pouring tasks, paving the way for more complex manipulations such as table-setting and drink serving.
Zoom password: 217944
Livestream Information
LivestreamMay 11, 2023 (Thursday) 10:30am
Meeting Password: 217944
Explore Similar Events
-
Loading Similar Events...