Presented By: Center for Connected and Automated Transportation
Safety Benchmarking of Automated Driving Systems: The Challenges of Apples to Apples Comparison
Carol Flannagan, Ph.D.
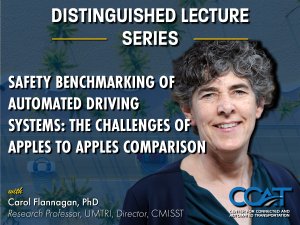
Current Automated Driving Systems (ADS) have reached the point of fully driverless deployments and even carrying passengers, so the question being asked is “Are ADS safer than humans?” This question turns out to be hard to answer because: 1) human-driver data systems were built to answer a different set of questions, and 2) ADS are being deployed in specific ODDs such that their crash rates may not be directly comparable to human-driver crash rates developed from national datasets. In this talk, Dr. Carol Flannagan will explore “Apples to Apples” safety comparisons between ADS and human drivers. What would the perfect comparison look like? What has been done? What are the challenges to achieving the perfect comparison and what can be done about it? Finally, what reasonable compromises can be made to prevent the perfect from becoming the enemy of the good?
---
About the speaker:
Dr. Flannagan has over 30 years of experience conducting data analysis and research on injury risk related to motor vehicle crashes. Her recent work on crash-avoidance technologies includes the application of statistical methods to estimate the effectiveness and potential benefits of crash-avoidance technologies as well as the safety assessment of automated driving systems (ADS). Dr. Flannagan’s work often involves the fusion and analysis of large state- and national-level databases, including crash, EMS, and hospital data. These fused databases are useful in analyzing the effect of a wide variety of countermeasures on crash involvement and injury risk. Finally, Dr. Flannagan has worked to develop and apply a wide variety of new and old statistical approaches, such as natural language processing, Bayesian methods, and causal inference methods to address transportation data issues and answer transportation research questions.
---
About the speaker:
Dr. Flannagan has over 30 years of experience conducting data analysis and research on injury risk related to motor vehicle crashes. Her recent work on crash-avoidance technologies includes the application of statistical methods to estimate the effectiveness and potential benefits of crash-avoidance technologies as well as the safety assessment of automated driving systems (ADS). Dr. Flannagan’s work often involves the fusion and analysis of large state- and national-level databases, including crash, EMS, and hospital data. These fused databases are useful in analyzing the effect of a wide variety of countermeasures on crash involvement and injury risk. Finally, Dr. Flannagan has worked to develop and apply a wide variety of new and old statistical approaches, such as natural language processing, Bayesian methods, and causal inference methods to address transportation data issues and answer transportation research questions.