Presented By: Department of Statistics
Statistics Department Seminar Series: Kabir Verchand, Gary C. Butler Family Postdoctoral Fellow, Georgia Institute of Technology and Cambridge University
"Estimation with missing data: Moving beyond Missing (Completely) at Random"
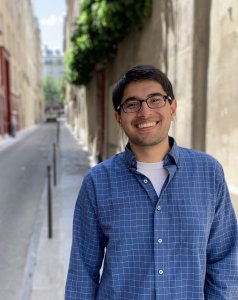
Abstract: Modern data pipelines are growing both in size and complexity, introducing tradeoffs across various aspects of the associated learning challenges. A key source of complexity, and the focus of this talk, is missing data. Estimators designed to handle missingness often rely on strong assumptions about the mechanism by which data is missing, such as that the data is missing completely at random (MCAR). By contrast, real data is rarely MCAR. In the absence of these strong assumptions, can we still trust these estimators?
In this talk, I will present a framework that bridges the gap between the MCAR and assumption-free settings. This framework reveals an inherent tradeoff between estimation accuracy and robustness to modeling assumptions. Focusing on the fundamental task of mean estimation, I will then present estimators which optimally navigate this tradeoff, offering both improved robustness and performance.
https://kabirverchand.github.io/
In this talk, I will present a framework that bridges the gap between the MCAR and assumption-free settings. This framework reveals an inherent tradeoff between estimation accuracy and robustness to modeling assumptions. Focusing on the fundamental task of mean estimation, I will then present estimators which optimally navigate this tradeoff, offering both improved robustness and performance.
https://kabirverchand.github.io/