Presented By: Department of Statistics
Dissertation Defense: Some Contributions to High Dimensional Mixed-Effects Logistic Regression Models
Jun Guo
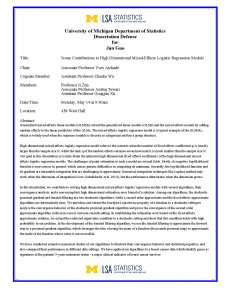
Generalized mixed-effects linear models (GLMMs) extend the generalized linear models (GLMs) and the mixed effects models by adding random effects to the linear predictors of the GLMs. The mixed-effects logistic regression model is a typical example of the GLMMs, which is widely used when the response variable is discrete or categorical and has a group structure.
High dimensional mixed-effects logistic regression model refers to the scenario when the number of fixed effects coefficients p is (much) larger than the sample size N, while the rank q of the random effects variance-covariance matrix is much smaller than the sample size N. Our goal in this dissertation is to infer about the unknown high dimensional fixed effects coefficients of the high dimensional mixed-effects logistic regression models. The challenges of point estimation in such a model are several folds. Firstly, its negative log-likelihood function is non-convex in general, which causes generic difficulties in computing its minimum. Secondly, the log-likelihood function and its gradient are intractable integration that are challenging to approximate. Numerical integration techniques like Laplace method may work when the dimension of integration is low (Schelldorfer et al. 2013), but the performance deteriorates when the dimension grows.
In this dissertation, we contribute to solving high dimensional mixed-effects logistic regression models with several algorithms, their convergence analysis, and a non-asymptotic high dimensional estimation error bound of a solution. Among our algorithms, the stochastic proximal gradient and iterated filtering are two stochastic algorithms, while a second order approximate and the fixed-effects approximate algorithms are deterministic ones. We introduce and extend the Kurdyka-Lojasiewicz property of a function to a stochastic setting to analyze the convergence behavior of the stochastic proximal gradient algorithm and prove the convergence of the second order approximate algorithm in the non-convex and non-smooth setting. In establishing the estimation error bound of the fixed-effects approximate solution, we extend the restricted eigenvalue condition to a stochastic setting and show that this condition holds with high probability in our problem. In the development of the iterated filtering algorithm, we use the iterated filtering to approximate the forward step in a proximal gradient algorithm, which leverages the idea of using the mean of a function (the pseudo proximal map) to approximate the mode of the function whose value is not accessible.
We have conducted extensive numerical studies of our algorithms to illustrate their convergence behavior and statistical properties, and also compared their performance in different data settings. We have applied our algorithms to a breast cancer data which identify genes as signatures of the patients' 5-years metastasis status - a major clinical indicator of breast cancer survivor.
High dimensional mixed-effects logistic regression model refers to the scenario when the number of fixed effects coefficients p is (much) larger than the sample size N, while the rank q of the random effects variance-covariance matrix is much smaller than the sample size N. Our goal in this dissertation is to infer about the unknown high dimensional fixed effects coefficients of the high dimensional mixed-effects logistic regression models. The challenges of point estimation in such a model are several folds. Firstly, its negative log-likelihood function is non-convex in general, which causes generic difficulties in computing its minimum. Secondly, the log-likelihood function and its gradient are intractable integration that are challenging to approximate. Numerical integration techniques like Laplace method may work when the dimension of integration is low (Schelldorfer et al. 2013), but the performance deteriorates when the dimension grows.
In this dissertation, we contribute to solving high dimensional mixed-effects logistic regression models with several algorithms, their convergence analysis, and a non-asymptotic high dimensional estimation error bound of a solution. Among our algorithms, the stochastic proximal gradient and iterated filtering are two stochastic algorithms, while a second order approximate and the fixed-effects approximate algorithms are deterministic ones. We introduce and extend the Kurdyka-Lojasiewicz property of a function to a stochastic setting to analyze the convergence behavior of the stochastic proximal gradient algorithm and prove the convergence of the second order approximate algorithm in the non-convex and non-smooth setting. In establishing the estimation error bound of the fixed-effects approximate solution, we extend the restricted eigenvalue condition to a stochastic setting and show that this condition holds with high probability in our problem. In the development of the iterated filtering algorithm, we use the iterated filtering to approximate the forward step in a proximal gradient algorithm, which leverages the idea of using the mean of a function (the pseudo proximal map) to approximate the mode of the function whose value is not accessible.
We have conducted extensive numerical studies of our algorithms to illustrate their convergence behavior and statistical properties, and also compared their performance in different data settings. We have applied our algorithms to a breast cancer data which identify genes as signatures of the patients' 5-years metastasis status - a major clinical indicator of breast cancer survivor.
Explore Similar Events
-
Loading Similar Events...