Presented By: Michigan Institute for Data Science
Computational Neuroscience, Time Complexity, and Spacetime Analytics
Ivo Dinov – Professor, HBBS/SoN, DCMB/SoM, MIDAS
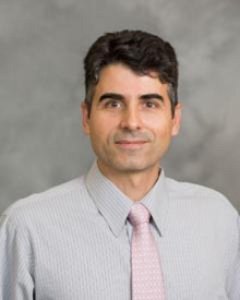
The proliferation of digital information in all human experiences presents difficult challenges and offers unique opportunities of managing, modeling, analyzing, interpreting, and visualizing heterogeneous data. There is a substantial need to develop, validate, productize, and support novel mathematical techniques, advanced statistical computing algorithms, transdisciplinary tools, and effective artificial intelligence apps.
Spacekime analytics is a new technique for modeling high-dimensional longitudinal data, such as functional magnetic resonance imaging (fMRI). This approach relies on extending the notions of time, events, particles, and wave functions to complex-time (kime), complex-events (kevents), data and inference-functions, respectively. This talk will illustrate how the kime-magnitude (longitudinal time order) and kime-direction (phase) affect the subsequent predictive analytics and the induced scientific inference. The mathematical foundation of spacekime calculus reveals various statistical implications including inferential uncertainty and a Bayesian formulation of spacekime analytics. Complexifying time allows the lifting of all commonly observed processes from the classical 4D Minkowski spacetime to a 5D spacetime manifold, where a number of interesting mathematical problems arise.
Spacekime analytics transforms time-varying data, such as time-series observations, into higher-dimensional manifolds representing complex-valued and kime-indexed surfaces (kime-surfaces). This process uncovers some of the intricate structure in high-dimensional data that may be intractable in the classical space-time representation of the data. In addition, the spacekime representation facilitates the development of innovative data science analytical methods for model-based and model-free scientific inference, derived computed phenotyping, and statistical forecasting. Direct neuroscience science applications of spacekime analytics will be demonstrated using simulated data and clinical observations (e.g., UK Biobank).
Spacekime analytics is a new technique for modeling high-dimensional longitudinal data, such as functional magnetic resonance imaging (fMRI). This approach relies on extending the notions of time, events, particles, and wave functions to complex-time (kime), complex-events (kevents), data and inference-functions, respectively. This talk will illustrate how the kime-magnitude (longitudinal time order) and kime-direction (phase) affect the subsequent predictive analytics and the induced scientific inference. The mathematical foundation of spacekime calculus reveals various statistical implications including inferential uncertainty and a Bayesian formulation of spacekime analytics. Complexifying time allows the lifting of all commonly observed processes from the classical 4D Minkowski spacetime to a 5D spacetime manifold, where a number of interesting mathematical problems arise.
Spacekime analytics transforms time-varying data, such as time-series observations, into higher-dimensional manifolds representing complex-valued and kime-indexed surfaces (kime-surfaces). This process uncovers some of the intricate structure in high-dimensional data that may be intractable in the classical space-time representation of the data. In addition, the spacekime representation facilitates the development of innovative data science analytical methods for model-based and model-free scientific inference, derived computed phenotyping, and statistical forecasting. Direct neuroscience science applications of spacekime analytics will be demonstrated using simulated data and clinical observations (e.g., UK Biobank).
Explore Similar Events
-
Loading Similar Events...