Presented By: Michigan Institute for Data Science
An end-to-end deep learning system for rapid analysis of the breath metabolome with applications in critical care illness and beyond
Christopher Gillies – Assistant Research Scientist, Emergency Medicine
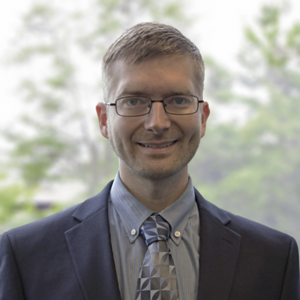
The metabolome is the set of low-molecular-weight metabolites and its quantification represents a summary of the physiological state of an organism. Metabolite concentration levels in biospecimens are important for many critical care health illnesses like sepsis and acute respiratory distress syndrome (ARDS). Sepsis is responsible for 35% of patients who die in the hospital and ARDS has a mortality rate of 40%. Missing data is a common challenge in metabolomics datasets. Many metabolomics investigators impute fixed values for missing metabolite concentrations and this imputation approach leads to lower statistical power, biased parameter estimates, and reduced prediction accuracy. Certain applications of metabolomics data, like breath analysis by gas chromatography, used for the prediction or detection of ARDS, can be done without the quantification of individual metabolites. This would circumvent the quantification step of individual metabolites, eliminating the missing data problem. Our team has developed a rapid gas chromatography breath analyzer, which has been challenged by missing data, a time-consuming process of breath signature alignment, and the following quantification of metabolites across patients. Analyzing the breath signal directly could eliminate these challenges. End-to-end deep learning systems are neural networks that operate directly on a raw data source and make a prediction directly for the target application. These systems have been successful in diverse fields from speech recognition to medicine. We envision an end-to-end deep learning that leverages transfer learning, from the collection of many healthy samples, that could rapidly multiply the applications of our breath analyzer. The end-to-end deep learning system will enhance our breath analyzer so it could be used more efficiently in areas of the intensive care unit to the battlefield to identity patients or soldiers with critical illnesses like sepsis and ARDS and monitor longitudinal changes in breath metabolites.
Explore Similar Events
-
Loading Similar Events...