Presented By: Michigan Institute for Data Science
Machine learning-guided equations for the on-demand prediction of natural gas storage capacities of materials for vehicular applications
Alauddin Ahmed – Assistant Research Scientist, Mechanical Engineering
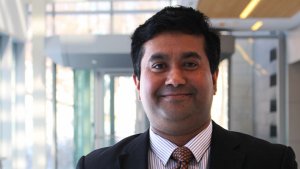
Transportation is responsible for nearly one-third of the world’s carbon dioxide (CO2) emission because of burning fossil fuel. While we dream for zero-carbon vehicles, future projections suggest little decline in fossil fuel consumption by the transportation sector until 2050. Therefore, ‘bending the curve’ of CO2 emission prompts the adoption of low-cost and reduced-emission alternative fuels. Natural gas (NG), the most abundant fossil fuel on earth, is such an alternative with nearly 25% lower carbon footprint and lower price compared to its gasoline counterpart. However, the widespread adoption of natural gas as a vehicular fuel is hindered by the scarcity of high-capacity, light-weight, low-cost, and safe storage systems. Recently, materials-based natural gas storage for vehicular applications have become one of the most viable options. Especially, nanoporous materials (NPMs) are in the spotlight of the U.S. Department of Energy (DOE) because of their exceptional energy storage capacities. However, the number of such NPMs is nearly infinite. It is unknown, a priori, which materials would have the expected natural gas storage capacity. Therefore, searching a high-performing material is like ‘finding a needle in a haystack’ that slows down the speed of materials discovery against growing technological demand. Here we present a novel approach of developing machine learning-guided equations for the on-demand prediction of energy storage capacities of NPMs using a few physically meaningful structural properties. These equations provide users the ability to calculate energy storage capacity of an arbitrary NPM rapidly using only paper and pencil. We show the utility of these equations by predicting NG storage of over 500,000 covalent-organic frameworks (COFs), a class of NPMs. We discovered a COF with record-setting NG storage capacity, surpassing the unmet target set by DOE. In principle, the data-driven approach presented here might be relevant to other disciplines including science, engineering, and health care.
Explore Similar Events
-
Loading Similar Events...