Presented By: Michigan Institute for Data Science
Fusing Computer Vision And Space Weather Modeling
David Fouhey – Assistant Professor, UM EECS
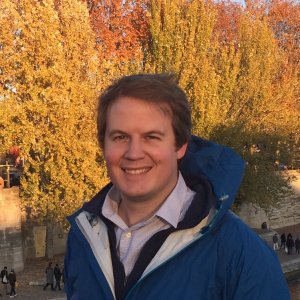
Space weather has impacts on Earth ranging from rare, immensely disruptive events (e.g., electrical blackouts caused by solar flares and coronal mass ejections) to more frequent impacts (e.g., satellite GPS interference from fluctuations in the Earth’s ionosphere caused by rapid variations in the solar extreme UV emission). Earth-impacting events are driven by changes in the Sun’s magnetic field; we now have myriad instruments capturing petabytes worth of images of the Sun at a variety of wavelengths, resolutions, and vantage points. These data present opportunities for learning-based computer vision since the massive, well-calibrated image archive is often accompanied by physical models. This talk will describe some of the work that we have been doing to start integrating computer vision and space physics by learning mappings from one image or representation of the Sun to another. I will center the talk on a new system we have developed that emulates parts of the data processing pipeline of the Solar Dynamics Observatory’s Helioseismic and Magnetic Imager (SDO/HMI). This pipeline produces data products that help study and serve as boundary conditions for solar models of the energetic events alluded to above. Our deep-learning-based system emulates a key component hundreds of times faster than the current method, potentially opening doors to new applications in near-real-time space weather modeling. In keeping with the goals of the symposium, however, I will focus on some of the benefits close collaboration has enabled in terms of understanding how to frame the problem, measure success of the model, and even set up the deep network.
Explore Similar Events
-
Loading Similar Events...