Presented By: Michigan Robotics
Robotic Manipulation under Transparency and Translucency from Light-field Sensing
Robotics PhD Defense, Zheming Zhou
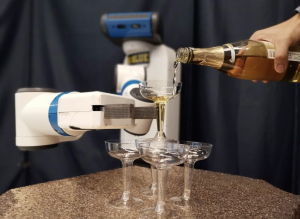
From frosted windows to plastic containers to refractive fluids, transparency and translucency are prevalent in human environments. The material properties of translucent objects challenge many of our assumptions in robotic perception. For example, the most common RGB-D sensors in robotic applications require the sensing of an infrared structured pattern from a Lambertian reflectance of surfaces. As such, transparent and translucent objects often remain invisible to robot perception. Thus, introducing methods that would enable robots to correctly perceive and then interact with the environment would be highly beneficial. Light-field (or plenoptic) cameras, for instance, which carry light direction and intensity, make it possible to perceive visual clues on object surfaces from reflection and refraction. In this dissertation, we explore the inference of transparent and translucent objects from plenoptic observations for robotic perception and manipulation. We propose a novel plenoptic descriptor, Depth Likelihood Volume (DLV), that incorporates multi-view plenoptic observations to represent depth. The depth of a pixel is then represented as a distribution rather than a single value. Through the DLV distribution, we can infer the layered translucency structure of the scene with transparent and translucent objects for robot manipulation tasks. Building on the DLV, we present the Plenoptic Monte Carlo Localization algorithm, PMCL, as a generative method to infer 6-DoF poses of objects in settings with translucency. PMCL is able to localize both isolated transparent objects and opaque objects behind translucent objects using a previously computed DLV. We evaluate PMCL by comparing x estimated poses against ground-truth poses and demonstrate the use of these pose estimates for object pick and place tasks. The uncertainty induced by transparency and translucency for pose estimation increases greatly as scenes become more cluttered. However, robot grasping does not necessarily require estimation of 6-DoF object poses. Given multi-view plenoptic observations, we propose GlassLoc to localize feasible grasp poses over a pile of transparent objects. In GlassLoc, a convolutional neural network is introduced to learn DLV features for classifying grasp poses with grasping confidence. GlassLoc also suppresses the reflectance by checking pixel consistency over multi-view plenoptic observations, which leads to more stable DLV representation. We evaluate GlassLoc in the context of a pick-and-place task for transparent tableware in a cluttered tabletop environment. We further observe that the transparent and translucent objects will generate distinguishable features in the light-field epipolar image plane, which provides information about the object location. With this insight, we propose Light-field Inference of Transparency, LIT, as a two-stage generative-discriminative refractive object localization approach. In the discriminative stage, LIT uses convolutional neural networks to learn reflection and distortion features from photorealistic-rendered light-field images. The learned features guide generative object location inference through local depth estimation and particle optimization. With the LIT pipeline, we also create the light-field dataset for the task of transparent objects recognition, segmentation, and pose estimation. We compare LIT with three state-of-the-art pose estimators to show our efficacy in the transparent object localization task. We also perform a robot demonstration by picking champagne cups up from a textureless table and building a champagne tower using the LIT pipeline.
Explore Similar Events
-
Loading Similar Events...