Presented By: Michigan Program in Survey and Data Science
Michael Elliott - Combining Probability Non-probability Samples - JPSM MPSDS Seminar Series
Michigan Program in Survey and Data Science and the Joint Program in Survey Methodology Seminar Series
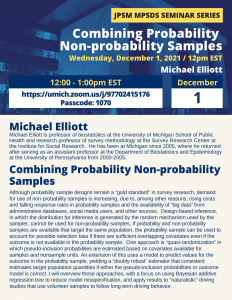
Michael Elliott is professor of biostatistics at the University of Michigan School of Public Health and research professor of survey methodology at the Survey Research Center at the Institute for Social Research. He has been at Michigan since 2005, where he returned after serving as an assistant professor at the Department of Biostatistics and Epidemiology at the University of Pennsylvania from 2000-2005.
COMBINING PROBABILITY NON-PROBABILITY SAMPLES
Although probability sample designs remain a “gold standard” in survey research, demand for use of non-probability samples is increasing, due to, among other reasons, rising costs and falling response rates in probability samples and the availability of “big data” from administrative databases, social media users, and other sources. Design-based inference, in which the distribution for inference is generated by the random mechanism used by the sampler, cannot be used for non-probability samples. If probability and non-probability samples are available that target the same population, the probability sample can be used to account for possible selection bias if there are sufficient overlapping covariates even if the outcome is not available in the probability sample. One approach is “quasi-randomization” in which pseudo-inclusion probabilities are estimated based on covariates available for samples and nonsample units. An extension of this uses a model to predict values for the outcome in the probability sample, yielding a “doubly robust” estimator that consistent estimates target population quantities if either the pseudo-inclusion probabilities or outcome model is correct. I will overview these approaches, with a focus on using Bayesian additive regression tree to reduce model misspecification, and apply results to “naturalistic” driving studies that use volunteer samples to follow long-term driving behavior.
COMBINING PROBABILITY NON-PROBABILITY SAMPLES
Although probability sample designs remain a “gold standard” in survey research, demand for use of non-probability samples is increasing, due to, among other reasons, rising costs and falling response rates in probability samples and the availability of “big data” from administrative databases, social media users, and other sources. Design-based inference, in which the distribution for inference is generated by the random mechanism used by the sampler, cannot be used for non-probability samples. If probability and non-probability samples are available that target the same population, the probability sample can be used to account for possible selection bias if there are sufficient overlapping covariates even if the outcome is not available in the probability sample. One approach is “quasi-randomization” in which pseudo-inclusion probabilities are estimated based on covariates available for samples and nonsample units. An extension of this uses a model to predict values for the outcome in the probability sample, yielding a “doubly robust” estimator that consistent estimates target population quantities if either the pseudo-inclusion probabilities or outcome model is correct. I will overview these approaches, with a focus on using Bayesian additive regression tree to reduce model misspecification, and apply results to “naturalistic” driving studies that use volunteer samples to follow long-term driving behavior.
Livestream Information
ZoomDecember 1, 2021 (Wednesday) 12:00pm
Meeting ID: 97702415176
Meeting Password: 1070
Explore Similar Events
-
Loading Similar Events...