Presented By: Department of Economics
Econometrics: Adversarial machine learning and instrumental variables for flexible causal modeling
Vasilis Syrgkanis, Microsoft Research
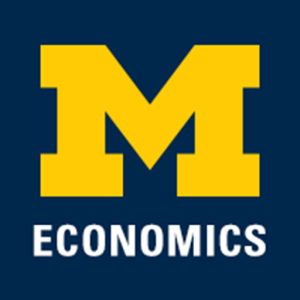
Abstract:
Machine learning models are increasingly being used to automate decision-making in a multitude of domains. Making good decisions requires uncovering causal relationships from data. Many causal estimation problems reduce to estimating a model that satisfies a set of conditional moment restrictions. We develop an approach for estimating flexible models defined via conditional moment restrictions, with a prototypical application being non-parametric instrumental variable regression. We introduce a min-max criterion function, under which the estimation problem can be thought of as solving a zero-sum game between a modeler who is optimizing over the hypothesis space of the target causal model and an adversary who identifies violating moments over a test function space. We analyze the statistical estimation rate of the resulting estimator for arbitrary hypothesis spaces, with respect to an appropriate analogue of the mean squared error metric, for ill-posed inverse problems. We show that when the minimax criterion is regularized with a second moment penalty on the test function and the test function space is sufficiently rich, then the estimation rate scales with the critical radius of the hypothesis and test function spaces, a quantity which typically gives tight fast rates. Our main result follows from a novel localized Rademacher analysis of statistical learning problems defined via minimax objectives. We provide applications of our main results for several hypothesis spaces used in practice such as: reproducing kernel Hilbert spaces, high dimensional sparse linear functions, spaces defined via shape constraints, ensemble estimators such as random forests, and neural networks. For each of these applications we provide computationally efficient optimization methods for solving the corresponding minimax problem and stochastic first-order heuristics for neural networks.
Based on joint works with: Nishanth Dikkala, Greg Lewis and Lester Mackey
*To join the seminar, please contact: econometrics-seminar-requests@umich.edu
Machine learning models are increasingly being used to automate decision-making in a multitude of domains. Making good decisions requires uncovering causal relationships from data. Many causal estimation problems reduce to estimating a model that satisfies a set of conditional moment restrictions. We develop an approach for estimating flexible models defined via conditional moment restrictions, with a prototypical application being non-parametric instrumental variable regression. We introduce a min-max criterion function, under which the estimation problem can be thought of as solving a zero-sum game between a modeler who is optimizing over the hypothesis space of the target causal model and an adversary who identifies violating moments over a test function space. We analyze the statistical estimation rate of the resulting estimator for arbitrary hypothesis spaces, with respect to an appropriate analogue of the mean squared error metric, for ill-posed inverse problems. We show that when the minimax criterion is regularized with a second moment penalty on the test function and the test function space is sufficiently rich, then the estimation rate scales with the critical radius of the hypothesis and test function spaces, a quantity which typically gives tight fast rates. Our main result follows from a novel localized Rademacher analysis of statistical learning problems defined via minimax objectives. We provide applications of our main results for several hypothesis spaces used in practice such as: reproducing kernel Hilbert spaces, high dimensional sparse linear functions, spaces defined via shape constraints, ensemble estimators such as random forests, and neural networks. For each of these applications we provide computationally efficient optimization methods for solving the corresponding minimax problem and stochastic first-order heuristics for neural networks.
Based on joint works with: Nishanth Dikkala, Greg Lewis and Lester Mackey
*To join the seminar, please contact: econometrics-seminar-requests@umich.edu
Livestream Information
LivestreamDecember 2, 2021 (Thursday) 2:30pm
Joining Information Not Yet Available
Explore Similar Events
-
Loading Similar Events...