Presented By: Industrial & Operations Engineering
Wilbert Steffy Lectureship with Jeff Wu
Spatial-temporal kriging and Navier-Stokes equations: a prominent example of engineering analytics
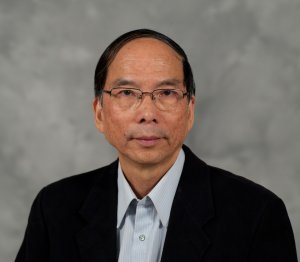
The Wilbert Steffy Lectureship was established in 2003 to honor one of Industrial and Operations Engineering’s early distinguished faculty, Wilbert Steffy. Jeff Wu, the Coca-Cola Chair in Engineering Statistics and Professor in the H. Milton School of Industrial and Systems Engineering at Georgia Tech, is our distinguished speaker this year.
Lurie Engineering Center (Johnson Rooms)
Thursday, November 3, 3 - 4 pm ET
Reception to follow with hors d'oeuvres.
Speaker Bio: C. F. Jeff Wu is Professor and Coca Cola Chair in Engineering Statistics at the School of Industrial and Systems Engineering, Georgia Institute of Technology. He was elected a Member of the National Academy of Engineering (2004), and a Member (Academician) of Academia Sinica (2000). A Fellow of American Society for Quality, of Institute of Mathematical Statistics, of INFORMS, and of American Statistical Association. He received the COPSS Presidents’ Award in 1987, COPSS Fisher Lecture Award in 2011, Deming Lecture in 2012. He has won other awards, including the Shewhart Medal (2008), the Pan Wenyuan Technology Award (2008), Class of 1934 Distinguished Professor Award and Sigma Xi Monie A. Ferst Award both at Georgia Institute of Technology in 2020. He was the 1998 Mahalanobis Memorial Lecturer at the Indian Statistical Institutes, received the inaugural Akaike Memorial Lecture Award in 2016 sponsored by the Japan Statistical Society and the Institute of Statistical Mathematics, Tokyo, the 2017 Box Medal from ENBIS, and an honorary doctor degree at the University of Waterloo.
He has published more than 190 research articles. He has supervised 51 Ph.D.'s, out of which more than half are teaching in major research departments in statistics/engineering/business in US/Canada/Asia/Europe. Among them, there are 24 Fellows of ASA, IMS, ASQ, IAQ, and IIE, four are editors of major statistics journals, and one is a Fellow of Royal Society of Canada (FRSC). He co-authors with Mike Hamada the book "Experiments: Planning, Analysis, and Optimization" (Wiley, 3rd ed. 2021) and with R. Mukerjee the book “A Modern Theory of Factorial Designs” (Springer, 2006).
Abstract: Most “learning” in big data is driven by the data alone. Some people may believe this is sufficient because of the sheer data size. If the physical world is involved, this approach is often insufficient. In this talk I will give a recent study to illustrate how physics and data are used jointly to learn about the “truth” of the physical world. It also serves as an example of engineering analytics, which in itself has many forms and meanings. In an attempt to understand the turbulence behavior of an injector, a new design methodology is needed which combines engineering physics, computer simulations and statistical modeling. There are two key challenges: the simulation of high-fidelity spatial-temporal flows (using the Navier-Stokes equations) is computationally expensive, and the analysis and modeling of this data requires physical insights and statistical tools. A surrogate model is presented for efficient flow prediction in injectors with varying geometries, devices commonly used in many engineering applications. The novelty lies in incorporating properties of the fluid flow as simplifying model assumptions, which allows for quick emulation in practical turnaround times, and also reveals interesting flow physics which can guide further investigations.
Lurie Engineering Center (Johnson Rooms)
Thursday, November 3, 3 - 4 pm ET
Reception to follow with hors d'oeuvres.
Speaker Bio: C. F. Jeff Wu is Professor and Coca Cola Chair in Engineering Statistics at the School of Industrial and Systems Engineering, Georgia Institute of Technology. He was elected a Member of the National Academy of Engineering (2004), and a Member (Academician) of Academia Sinica (2000). A Fellow of American Society for Quality, of Institute of Mathematical Statistics, of INFORMS, and of American Statistical Association. He received the COPSS Presidents’ Award in 1987, COPSS Fisher Lecture Award in 2011, Deming Lecture in 2012. He has won other awards, including the Shewhart Medal (2008), the Pan Wenyuan Technology Award (2008), Class of 1934 Distinguished Professor Award and Sigma Xi Monie A. Ferst Award both at Georgia Institute of Technology in 2020. He was the 1998 Mahalanobis Memorial Lecturer at the Indian Statistical Institutes, received the inaugural Akaike Memorial Lecture Award in 2016 sponsored by the Japan Statistical Society and the Institute of Statistical Mathematics, Tokyo, the 2017 Box Medal from ENBIS, and an honorary doctor degree at the University of Waterloo.
He has published more than 190 research articles. He has supervised 51 Ph.D.'s, out of which more than half are teaching in major research departments in statistics/engineering/business in US/Canada/Asia/Europe. Among them, there are 24 Fellows of ASA, IMS, ASQ, IAQ, and IIE, four are editors of major statistics journals, and one is a Fellow of Royal Society of Canada (FRSC). He co-authors with Mike Hamada the book "Experiments: Planning, Analysis, and Optimization" (Wiley, 3rd ed. 2021) and with R. Mukerjee the book “A Modern Theory of Factorial Designs” (Springer, 2006).
Abstract: Most “learning” in big data is driven by the data alone. Some people may believe this is sufficient because of the sheer data size. If the physical world is involved, this approach is often insufficient. In this talk I will give a recent study to illustrate how physics and data are used jointly to learn about the “truth” of the physical world. It also serves as an example of engineering analytics, which in itself has many forms and meanings. In an attempt to understand the turbulence behavior of an injector, a new design methodology is needed which combines engineering physics, computer simulations and statistical modeling. There are two key challenges: the simulation of high-fidelity spatial-temporal flows (using the Navier-Stokes equations) is computationally expensive, and the analysis and modeling of this data requires physical insights and statistical tools. A surrogate model is presented for efficient flow prediction in injectors with varying geometries, devices commonly used in many engineering applications. The novelty lies in incorporating properties of the fluid flow as simplifying model assumptions, which allows for quick emulation in practical turnaround times, and also reveals interesting flow physics which can guide further investigations.
Explore Similar Events
-
Loading Similar Events...